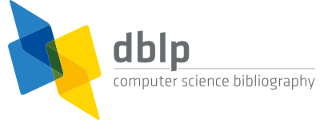


default search action
23rd MICCAI 2020: Lima, Peru - Challenges
- Nadya Shusharina
, Mattias P. Heinrich
, Ruobing Huang
:
Segmentation, Classification, and Registration of Multi-modality Medical Imaging Data - MICCAI 2020 Challenges, ABCs 2020, L2R 2020, TN-SCUI 2020, Held in Conjunction with MICCAI 2020, Lima, Peru, October 4-8, 2020, Proceedings. Lecture Notes in Computer Science 12587, Springer 2021, ISBN 978-3-030-71826-8
ABCs - Anatomical Brain Barriers to Cancer Spread: Segmentation from CT and MR Images
- Nadya Shusharina
, Thomas Bortfeld, Carlos Cardenas
, Brian De
, Kevin Diao
, Soleil Hernandez
, Yufei Liu
, Sean Maroongroge, Jonas Söderberg, Moaaz Soliman:
Cross-Modality Brain Structures Image Segmentation for the Radiotherapy Target Definition and Plan Optimization. 3-15 - Xiaoyang Zou
, Qi Dou:
Domain Knowledge Driven Multi-modal Segmentation of Anatomical Brain Barriers to Cancer Spread. 16-26 - Munan Ning, Cheng Bian
, Chenglang Yuan, Kai Ma, Yefeng Zheng:
Ensembled ResUnet for Anatomical Brain Barriers Segmentation. 27-33 - Huai Chen
, Dahong Qian, Weiping Liu, Hui Li, Lisheng Wang
:
An Enhanced Coarse-to-Fine Framework for the Segmentation of Clinical Target Volume. 34-39 - Marco Langhans, Tobias Fechter, Dimos Baltas, Harald Binder, Thomas Bortfeld:
Automatic Segmentation of Brain Structures for Treatment Planning Optimization and Target Volume Definition. 40-48 - Skylar S. Gay
, Cenji Yu
, Dong Joo Rhee
, Carlos Sjogreen
, Raymond P. Mumme
, Callistus M. Nguyen
, Tucker J. Netherton
, Carlos E. Cárdenas S.
, Laurence E. Court
:
A Bi-directional, Multi-modality Framework for Segmentation of Brain Structures. 49-57
L2R - Learn2Reg: Multitask and Multimodal 3D Medical Image Registration
- Tony C. W. Mok, Albert C. S. Chung:
Large Deformation Image Registration with Anatomy-Aware Laplacian Pyramid Networks. 61-67 - Lasse Hansen
, Mattias P. Heinrich
:
Discrete Unsupervised 3D Registration Methods for the Learn2Reg Challenge. 68-73 - Stephanie Häger
, Stefan Heldmann
, Alessa Hering
, Sven Kuckertz
, Annkristin Lange
:
Variable Fraunhofer MEVIS RegLib Comprehensively Applied to Learn2Reg Challenge. 74-79 - Niklas Gunnarsson
, Jens Sjölund
, Thomas B. Schön
:
Learning a Deformable Registration Pyramid. 80-86 - Théo Estienne, Maria Vakalopoulou, Enzo Battistella, Alexandre Carré, Théophraste Henry, Marvin Lerousseau, Charlotte Robert, Nikos Paragios, Eric Deutsch:
Deep Learning Based Registration Using Spatial Gradients and Noisy Segmentation Labels. 87-93 - Marek Wodzinski
:
Multi-step, Learning-Based, Semi-supervised Image Registration Algorithm. 94-99 - Constance Fourcade, Mathieu Rubeaux, Diana Mateus:
Using Elastix to Register Inhale/Exhale Intrasubject Thorax CT: A Unsupervised Baseline to the Task 2 of the Learn2Reg Challenge. 100-105
TN-SCUI - Thyroid Nodule Segmentation and Classification in Ultrasound Images
- Mingyu Wang, Chenglang Yuan, Dasheng Wu, Yinghou Zeng, Shaonan Zhong, Weibao Qiu:
Automatic Segmentation and Classification of Thyroid Nodules in Ultrasound Images with Convolutional Neural Networks. 109-115 - Huai Chen, Shaoli Song, Xiuying Wang, Renzhen Wang, Deyu Meng, Lisheng Wang:
LRTHR-Net: A Low-Resolution-to-High-Resolution Framework to Iteratively Refine the Segmentation of Thyroid Nodule in Ultrasound Images. 116-121 - Zhe Tang, Jianqiang Ma:
Coarse to Fine Ensemble Network for Thyroid Nodule Segmentation. 122-128 - Yiwen Zhang, Haoran Lai, Wei Yang:
Cascade UNet and CH-UNet for Thyroid Nodule Segmentation and Benign and Malignant Classification. 129-134 - Jintao Lu, Xi Ouyang, Tianjiao Liu, Dinggang Shen:
Identifying Thyroid Nodules in Ultrasound Images Through Segmentation-Guided Discriminative Localization. 135-144 - Xueda Shen, Xi Ouyang, Tianjiao Liu, Dinggang Shen:
Cascaded Networks for Thyroid Nodule Diagnosis from Ultrasound Images. 145-154

manage site settings
To protect your privacy, all features that rely on external API calls from your browser are turned off by default. You need to opt-in for them to become active. All settings here will be stored as cookies with your web browser. For more information see our F.A.Q.