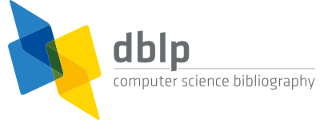


default search action
1st MLMIR@MICCAI 2018: Granada, Spain
- Florian Knoll
, Andreas K. Maier, Daniel Rueckert:
Machine Learning for Medical Image Reconstruction - First International Workshop, MLMIR 2018, Held in Conjunction with MICCAI 2018, Granada, Spain, September 16, 2018, Proceedings. Lecture Notes in Computer Science 11074, Springer 2018, ISBN 978-3-030-00128-5
Deep Learning for Magnetic Resonance Imaging
- Akshay Chaudhari
, Zhongnan Fang, Jin Hyung Lee, Garry Gold
, Brian A. Hargreaves:
Deep Learning Super-Resolution Enables Rapid Simultaneous Morphological and Quantitative Magnetic Resonance Imaging. 3-11 - Changheun Oh
, Dongchan Kim
, Jun-Young Chung, Yeji Han, HyunWook Park:
ETER-net: End to End MR Image Reconstruction Using Recurrent Neural Network. 12-20 - Ilkay Öksüz
, James R. Clough
, Aurélien Bustin
, Gastão Cruz
, Claudia Prieto
, René M. Botnar
, Daniel Rueckert, Julia A. Schnabel
, Andrew P. King:
Cardiac MR Motion Artefact Correction from K-space Using Deep Learning-Based Reconstruction. 21-29 - Muneer Ahmad Dedmari, Sailesh Conjeti, Santiago Estrada, Phillip Ehses, Tony Stöcker
, Martin Reuter:
Complex Fully Convolutional Neural Networks for MR Image Reconstruction. 30-38 - Fabian Balsiger
, Amaresha Shridhar Konar, Shivaprasad Chikop, Vimal Chandran, Olivier Scheidegger
, Sairam Geethanath, Mauricio Reyes:
Magnetic Resonance Fingerprinting Reconstruction via Spatiotemporal Convolutional Neural Networks. 39-46 - Eunju Cha
, Eung-Yeop Kim
, Jong Chul Ye
:
Improved Time-Resolved MRA Using k-Space Deep Learning. 47-54 - Chen Qin
, Wenjia Bai, Jo Schlemper, Steffen E. Petersen
, Stefan K. Piechnik, Stefan Neubauer, Daniel Rueckert:
Joint Motion Estimation and Segmentation from Undersampled Cardiac MR Image. 55-63 - Jo Schlemper, Daniel Coelho de Castro
, Wenjia Bai, Chen Qin
, Ozan Oktay, Jinming Duan
, Anthony N. Price, Joseph V. Hajnal
, Daniel Rueckert:
Bayesian Deep Learning for Accelerated MR Image Reconstruction. 64-71
Deep Learning for Computed Tomography
- Franz Thaler, Kerstin Hammernik
, Christian Payer
, Martin Urschler
, Darko Stern:
Sparse-View CT Reconstruction Using Wasserstein GANs. 75-82 - Bastian Bier, Katharina Aschoff, Christopher Syben, Mathias Unberath
, Marc E. Levenston
, Garry Gold
, Rebecca Fahrig, Andreas K. Maier:
Detecting Anatomical Landmarks for Motion Estimation in Weight-Bearing Imaging of Knees. 83-90 - Andreas Kofler, Markus Haltmeier, Christoph Kolbitsch, Marc Kachelrieß, Marc Dewey:
A U-Nets Cascade for Sparse View Computed Tomography. 91-99
Deep Learning for General Image Reconstruction
- Andreas Hauptmann
, Ben T. Cox
, Felix Lucka
, Nam Huynh, Marta M. Betcke, Paul C. Beard, Simon R. Arridge:
Approximate k-Space Models and Deep Learning for Fast Photoacoustic Reconstruction. 103-111 - Hanene Ben Yedder
, Aïcha BenTaieb, Majid Shokoufi, Amir Zahiremami, Farid Golnaraghi, Ghassan Hamarneh
:
Deep Learning Based Image Reconstruction for Diffuse Optical Tomography. 112-119 - Valeriy Vishnevskiy, Sergio J. Sanabria
, Orcun Goksel
:
Image Reconstruction via Variational Network for Real-Time Hand-Held Sound-Speed Imaging. 120-128 - Felix Horger, Tobias Würfl, Vincent Christlein, Andreas K. Maier:
Towards Arbitrary Noise Augmentation - Deep Learning for Sampling from Arbitrary Probability Distributions. 129-137 - Alon Baram, Moshe Safran, Avi Ben-Cohen, Hayit Greenspan:
Left Atria Reconstruction from a Series of Sparse Catheter Paths Using Neural Networks. 138-146 - Sanketh Vedula, Ortal Senouf, Grigoriy Zurakhov, Alexander M. Bronstein, Michael Zibulevsky, Oleg V. Michailovich, Dan Adam, Diana Gaitini:
High Quality Ultrasonic Multi-line Transmission Through Deep Learning. 147-155

manage site settings
To protect your privacy, all features that rely on external API calls from your browser are turned off by default. You need to opt-in for them to become active. All settings here will be stored as cookies with your web browser. For more information see our F.A.Q.