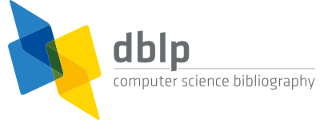


default search action
John P. Cunningham
Person information
- affiliation: Columbia University, Department of Statistics, New York, NY, USA
- affiliation: Washington University in St. Louis, Department of Electrical & Systems Engineering, MO, USA
- affiliation: University of Cambridge, Department of Engineering, UK
- affiliation (PhD 2009): Stanford University, Department of Electrical Engineering, CA, USA
Refine list

refinements active!
zoomed in on ?? of ?? records
view refined list in
export refined list as
2020 – today
- 2024
- [j16]Taiga Abe, Estefany Kelly Buchanan, Geoff Pleiss, John P. Cunningham:
Pathologies of Predictive Diversity in Deep Ensembles. Trans. Mach. Learn. Res. 2024 (2024) - [i35]Dan Biderman, Jose Javier Gonzalez Ortiz, Jacob Portes, Mansheej Paul, Philip Greengard, Connor Jennings, Daniel King, Sam Havens, Vitaliy Chiley, Jonathan Frankle, Cody Blakeney, John P. Cunningham:
LoRA Learns Less and Forgets Less. CoRR abs/2405.09673 (2024) - [i34]Natalie Maus, Kyurae Kim, Geoff Pleiss, David Eriksson, John P. Cunningham, Jacob R. Gardner:
Approximation-Aware Bayesian Optimization. CoRR abs/2406.04308 (2024) - [i33]Andrew Jesson, Nicolas Beltran-Velez, Quentin Chu, Sweta Karlekar, Jannik Kossen, Yarin Gal, John P. Cunningham, David M. Blei:
Estimating the Hallucination Rate of Generative AI. CoRR abs/2406.07457 (2024) - 2023
- [c52]Luhuan Wu, Brian L. Trippe, Christian A. Naesseth, David M. Blei, John P. Cunningham:
Practical and Asymptotically Exact Conditional Sampling in Diffusion Models. NeurIPS 2023 - [i32]Yixin Wang, David M. Blei, John P. Cunningham:
Posterior Collapse and Latent Variable Non-identifiability. CoRR abs/2301.00537 (2023) - [i31]Taiga Abe, Estefany Kelly Buchanan, Geoff Pleiss, John P. Cunningham:
Pathologies of Predictive Diversity in Deep Ensembles. CoRR abs/2302.00704 (2023) - [i30]Luhuan Wu, Brian L. Trippe, Christian A. Naesseth, David M. Blei, John P. Cunningham:
Practical and Asymptotically Exact Conditional Sampling in Diffusion Models. CoRR abs/2306.17775 (2023) - 2022
- [c51]Gabriel Loaiza-Ganem, Brendan Leigh Ross, Luhuan Wu, John P. Cunningham, Jesse C. Cresswell, Anthony L. Caterini:
Denoising Deep Generative Models. ICBINB 2022: 41-50 - [c50]Yao Fu, John P. Cunningham, Mirella Lapata:
Scaling Structured Inference with Randomization. ICML 2022: 6811-6828 - [c49]Jonathan Wenger, Geoff Pleiss, Philipp Hennig, John P. Cunningham, Jacob R. Gardner:
Preconditioning for Scalable Gaussian Process Hyperparameter Optimization. ICML 2022: 23751-23780 - [c48]Luhuan Wu, Geoff Pleiss, John P. Cunningham:
Variational nearest neighbor Gaussian process. ICML 2022: 24114-24130 - [c47]Taiga Abe, Estefany Kelly Buchanan, Geoff Pleiss, Richard S. Zemel, John P. Cunningham:
Deep Ensembles Work, But Are They Necessary? NeurIPS 2022 - [c46]Elliott Gordon-Rodríguez, Thomas P. Quinn, John P. Cunningham:
Data Augmentation for Compositional Data: Advancing Predictive Models of the Microbiome. NeurIPS 2022 - [c45]Jonathan Wenger, Geoff Pleiss, Marvin Pförtner, Philipp Hennig, John P. Cunningham:
Posterior and Computational Uncertainty in Gaussian Processes. NeurIPS 2022 - [i29]Luhuan Wu, Geoff Pleiss, John P. Cunningham:
Variational Nearest Neighbor Gaussian Processes. CoRR abs/2202.01694 (2022) - [i28]Taiga Abe, Estefany Kelly Buchanan, Geoff Pleiss, Richard S. Zemel, John P. Cunningham:
Deep Ensembles Work, But Are They Necessary? CoRR abs/2202.06985 (2022) - [i27]Elliott Gordon-Rodríguez, Gabriel Loaiza-Ganem, Andres Potapczynski, John P. Cunningham:
On the Normalizing Constant of the Continuous Categorical Distribution. CoRR abs/2204.13290 (2022) - [i26]Elliott Gordon-Rodríguez, Thomas P. Quinn, John P. Cunningham:
Data Augmentation for Compositional Data: Advancing Predictive Models of the Microbiome. CoRR abs/2205.09906 (2022) - [i25]Jonathan Wenger, Geoff Pleiss, Marvin Pförtner, Philipp Hennig, John P. Cunningham:
Posterior and Computational Uncertainty in Gaussian Processes. CoRR abs/2205.15449 (2022) - [i24]Gabriel Loaiza-Ganem, Brendan Leigh Ross, Luhuan Wu, John P. Cunningham, Jesse C. Cresswell, Anthony L. Caterini:
Denoising Deep Generative Models. CoRR abs/2212.01265 (2022) - 2021
- [j15]Elliott Gordon-Rodríguez
, Thomas P. Quinn
, John P. Cunningham:
Learning sparse log-ratios for high-throughput sequencing data. Bioinform. 38(1): 157-163 (2021) - [j14]Jackson Loper, David M. Blei, John P. Cunningham, Liam Paninski:
A general linear-time inference method for Gaussian Processes on one dimension. J. Mach. Learn. Res. 22: 234:1-234:36 (2021) - [j13]Rohan Shad
, John P. Cunningham, Euan A. Ashley, Curtis P. Langlotz
, William Hiesinger
:
Designing clinically translatable artificial intelligence systems for high-dimensional medical imaging. Nat. Mach. Intell. 3(11): 929-935 (2021) - [j12]Matthew R. Whiteway
, Dan Biderman
, Yoni Friedman
, Mario Dipoppa
, Estefany Kelly Buchanan
, Anqi Wu
, John Zhou
, Niccolò Bonacchi
, Nathaniel J. Miska
, Jean-Paul Noel
, Erica Rodriguez
, Michael Schartner
, Karolina Socha
, Anne E. Urai
, C. Daniel Salzman, International Brain Laboratory, John P. Cunningham, Liam Paninski:
Partitioning variability in animal behavioral videos using semi-supervised variational autoencoders. PLoS Comput. Biol. 17(9) (2021) - [c44]Luhuan Wu, Andrew Miller, Lauren Anderson, Geoff Pleiss, David M. Blei, John P. Cunningham:
Hierarchical Inducing Point Gaussian Process for Inter-domian Observations. AISTATS 2021: 2926-2934 - [c43]Andres Potapczynski, Luhuan Wu, Dan Biderman, Geoff Pleiss, John P. Cunningham:
Bias-Free Scalable Gaussian Processes via Randomized Truncations. ICML 2021: 8609-8619 - [c42]Geoff Pleiss, John P. Cunningham:
The Limitations of Large Width in Neural Networks: A Deep Gaussian Process Perspective. NeurIPS 2021: 3349-3363 - [c41]Yixin Wang, David M. Blei, John P. Cunningham:
Posterior Collapse and Latent Variable Non-identifiability. NeurIPS 2021: 5443-5455 - [c40]Anthony L. Caterini, Gabriel Loaiza-Ganem, Geoff Pleiss, John P. Cunningham:
Rectangular Flows for Manifold Learning. NeurIPS 2021: 30228-30241 - [i23]Andres Potapczynski, Luhuan Wu, Dan Biderman, Geoff Pleiss, John P. Cunningham:
Bias-Free Scalable Gaussian Processes via Randomized Truncations. CoRR abs/2102.06695 (2021) - [i22]Rohan Shad
, Nicolas Quach, Robyn Fong, Patpilai Kasinpila, Cayley Bowles, Miguel Castro, Ashrith Guha, Eddie Suarez, Stefan Jovinge, Sangjin Lee, Theodore Boeve, Myriam Amsallem, Xiu Tang, Francois Haddad, Yasuhiro Shudo, Y. Joseph Woo, Jeffrey J. Teuteberg, John P. Cunningham, Curtis P. Langlotz, William Hiesinger:
Predicting post-operative right ventricular failure using video-based deep learning. CoRR abs/2103.00364 (2021) - [i21]Luhuan Wu, Andrew Miller, Lauren Anderson
, Geoff Pleiss, David M. Blei, John P. Cunningham:
Hierarchical Inducing Point Gaussian Process for Inter-domain Observations. CoRR abs/2103.00393 (2021) - [i20]Rohan Shad
, John P. Cunningham, Euan A. Ashley, Curtis P. Langlotz, William Hiesinger:
Medical Imaging and Machine Learning. CoRR abs/2103.01938 (2021) - [i19]Rohan Shad, Nicolas Quach, Robyn Fong, Patpilai Kasinpila, Cayley Bowles, Kate M. Callon, Michelle C. Li, Jeffrey J. Teuteberg, John P. Cunningham, Curtis P. Langlotz, William Hiesinger:
Simulating time to event prediction with spatiotemporal echocardiography deep learning. CoRR abs/2103.02583 (2021) - [i18]Anthony L. Caterini, Gabriel Loaiza-Ganem, Geoff Pleiss, John P. Cunningham:
Rectangular Flows for Manifold Learning. CoRR abs/2106.01413 (2021) - [i17]Geoff Pleiss, John P. Cunningham:
The Limitations of Large Width in Neural Networks: A Deep Gaussian Process Perspective. CoRR abs/2106.06529 (2021) - [i16]Jonathan Wenger, Geoff Pleiss, Philipp Hennig, John P. Cunningham, Jacob R. Gardner:
Reducing the Variance of Gaussian Process Hyperparameter Optimization with Preconditioning. CoRR abs/2107.00243 (2021) - 2020
- [j11]Aki Vehtari, Andrew Gelman, Tuomas Sivula, Pasi Jylänki, Dustin Tran, Swupnil Sahai, Paul Blomstedt, John P. Cunningham, David Schiminovich, Christian P. Robert:
Expectation Propagation as a Way of Life: A Framework for Bayesian Inference on Partitioned Data. J. Mach. Learn. Res. 21: 17:1-17:53 (2020) - [c39]Elliott Gordon-Rodríguez, Gabriel Loaiza-Ganem, Geoff Pleiss, John P. Cunningham:
Uses and Abuses of the Cross-Entropy Loss: Case Studies in Modern Deep Learning. ICBINB@NeurIPS 2020: 1-10 - [c38]Elliott Gordon-Rodríguez, Gabriel Loaiza-Ganem, John P. Cunningham:
The continuous categorical: a novel simplex-valued exponential family. ICML 2020: 3637-3647 - [c37]Joshua I. Glaser, Matthew R. Whiteway, John P. Cunningham, Liam Paninski, Scott W. Linderman:
Recurrent Switching Dynamical Systems Models for Multiple Interacting Neural Populations. NeurIPS 2020 - [c36]Andres Potapczynski, Gabriel Loaiza-Ganem, John P. Cunningham:
Invertible Gaussian Reparameterization: Revisiting the Gumbel-Softmax. NeurIPS 2020 - [c35]Anqi Wu, Estefany Kelly Buchanan, Matthew R. Whiteway, Michael Schartner, Guido Meijer, Jean-Paul Noel, Erica Rodriguez, Claire Everett, Amy Norovich, Evan Schaffer, Neeli Mishra, C. Daniel Salzman, Dora E. Angelaki, Andrés Bendesky, International Brain Laboratory, John P. Cunningham, Liam Paninski:
Deep Graph Pose: a semi-supervised deep graphical model for improved animal pose tracking. NeurIPS 2020 - [i15]Yao Fu, Yansong Feng, John P. Cunningham:
Paraphrase Generation with Latent Bag of Words. CoRR abs/2001.01941 (2020) - [i14]Elliott Gordon-Rodríguez, Gabriel Loaiza-Ganem, John P. Cunningham:
The continuous categorical: a novel simplex-valued exponential family. CoRR abs/2002.08563 (2020) - [i13]Jackson Loper, David M. Blei, John P. Cunningham, Liam Paninski:
General linear-time inference for Gaussian Processes on one dimension. CoRR abs/2003.05554 (2020) - [i12]Elliott Gordon-Rodríguez, Gabriel Loaiza-Ganem, Geoff Pleiss, John P. Cunningham:
Uses and Abuses of the Cross-Entropy Loss: Case Studies in Modern Deep Learning. CoRR abs/2011.05231 (2020)
2010 – 2019
- 2019
- [c34]Gia-Lac Tran, Edwin V. Bonilla, John P. Cunningham, Pietro Michiardi, Maurizio Filippone:
Calibrating Deep Convolutional Gaussian Processes. AISTATS 2019: 1554-1563 - [c33]Gabriel Loaiza-Ganem, John P. Cunningham:
Deep Random Splines for Point Process Intensity Estimation. DGS@ICLR 2019 - [c32]Andrew C. Miller, Ziad Obermeyer, John P. Cunningham, Sendhil Mullainathan:
Discriminative Regularization for Latent Variable Models with Applications to Electrocardiography. ICML 2019: 4585-4594 - [c31]Gabriel Loaiza-Ganem, John P. Cunningham:
The continuous Bernoulli: fixing a pervasive error in variational autoencoders. NeurIPS 2019: 13266-13276 - [c30]Gabriel Loaiza-Ganem, Sean Perkins, Karen Schroeder, Mark M. Churchland, John P. Cunningham:
Deep Random Splines for Point Process Intensity Estimation of Neural Population Data. NeurIPS 2019: 13346-13356 - [c29]Yao Fu, Yansong Feng, John P. Cunningham:
Paraphrase Generation with Latent Bag of Words. NeurIPS 2019: 13623-13634 - [c28]Eleanor Batty, Matthew R. Whiteway, Shreya Saxena, Dan Biderman, Taiga Abe, Simon Musall, Winthrop Gillis, Jeffrey E. Markowitz, Anne Churchland, John P. Cunningham, Sandeep R. Datta, Scott W. Linderman, Liam Paninski:
BehaveNet: nonlinear embedding and Bayesian neural decoding of behavioral videos. NeurIPS 2019: 15680-15691 - [i11]Gabriel Loaiza-Ganem, John P. Cunningham:
Deep Random Splines for Point Process Intensity Estimation. CoRR abs/1903.02610 (2019) - [i10]Sean R. Bittner, John P. Cunningham:
Approximating exponential family models (not single distributions) with a two-network architecture. CoRR abs/1903.07515 (2019) - [i9]Gabriel Loaiza-Ganem, John P. Cunningham:
The continuous Bernoulli: fixing a pervasive error in variational autoencoders. CoRR abs/1907.06845 (2019) - [i8]Andres Potapczynski, Gabriel Loaiza-Ganem, John P. Cunningham:
Invertible Gaussian Reparameterization: Revisiting the Gumbel-Softmax. CoRR abs/1912.09588 (2019) - 2018
- [c27]Scott W. Linderman, Gonzalo E. Mena, Hal James Cooper, Liam Paninski, John P. Cunningham:
Reparameterizing the Birkhoff Polytope for Variational Permutation Inference. AISTATS 2018: 1618-1627 - [i7]Andrea Insabato, John P. Cunningham, Matthieu Gilson:
Bayesian estimation for large scale multivariate Ornstein-Uhlenbeck model of brain connectivity. CoRR abs/1805.10050 (2018) - [i6]Gia-Lac Tran, Edwin V. Bonilla, John P. Cunningham, Pietro Michiardi, Maurizio Filippone:
Calibrating Deep Convolutional Gaussian Processes. CoRR abs/1805.10522 (2018) - [i5]Daniel Hernández, Antonio Khalil Moretti, Ziqiang Wei
, Shreya Saxena, John P. Cunningham, Liam Paninski:
A Novel Variational Family for Hidden Nonlinear Markov Models. CoRR abs/1811.02459 (2018) - [i4]Andrew C. Miller, Ziad Obermeyer, David M. Blei, John P. Cunningham, Sendhil Mullainathan:
A Probabilistic Model of Cardiac Physiology and Electrocardiograms. CoRR abs/1812.00209 (2018) - 2017
- [j10]Stephan Mandt
, Florian Wenzel, Shinichi Nakajima, John P. Cunningham, Christoph Lippert, Marius Kloft:
Sparse probit linear mixed model. Mach. Learn. 106(9-10): 1621-1642 (2017) - [j9]Gonzalo E. Mena
, Lauren E. Grosberg, Sasidhar Madugula, Pawel Hottowy, Alan M. Litke, John P. Cunningham, E. J. Chichilnisky, Liam Paninski:
Electrical stimulus artifact cancellation and neural spike detection on large multi-electrode arrays. PLoS Comput. Biol. 13(11) (2017) - [c26]Francois Fagan, Jalaj Bhandari, John P. Cunningham:
Annular Augmentation Sampling. AISTATS 2017: 139-147 - [c25]Gabriel Loaiza-Ganem, Yuanjun Gao, John P. Cunningham:
Maximum Entropy Flow Networks. ICLR (Poster) 2017 - 2016
- [j8]Josh Merel, David E. Carlson
, Liam Paninski, John P. Cunningham:
Neuroprosthetic Decoder Training as Imitation Learning. PLoS Comput. Biol. 12(5) (2016) - [j7]Jeffrey S. Seely
, Matthew T. Kaufman
, Stephen I. Ryu, Krishna V. Shenoy, John P. Cunningham, Mark M. Churchland:
Tensor Analysis Reveals Distinct Population Structure that Parallels the Different Computational Roles of Areas M1 and V1. PLoS Comput. Biol. 12(11) (2016) - [c24]Kurt Cutajar, Michael A. Osborne, John P. Cunningham, Maurizio Filippone:
Preconditioning Kernel Matrices. ICML 2016: 2529-2538 - [c23]Benjamin Bloem-Reddy, John P. Cunningham:
Slice Sampling on Hamiltonian Trajectories. ICML 2016: 3050-3058 - [c22]Yuanjun Gao, Evan W. Archer, Liam Paninski, John P. Cunningham:
Linear dynamical neural population models through nonlinear embeddings. NIPS 2016: 163-171 - [c21]Uygar Sümbül, Douglas H. Roossien, Dawen Cai, Fei Chen, Nicholas Barry, John P. Cunningham, Edward S. Boyden, Liam Paninski:
Automated scalable segmentation of neurons from multispectral images. NIPS 2016: 1912-1920 - [c20]Francois Fagan, Jalaj Bhandari, John P. Cunningham:
Elliptical Slice Sampling with Expectation Propagation. UAI 2016 - [c19]Seth R. Flaxman, Dino Sejdinovic, John P. Cunningham, Sarah Filippi:
Bayesian Learning of Kernel Embeddings. UAI 2016 - 2015
- [j6]John P. Cunningham, Zoubin Ghahramani:
Linear dimensionality reduction: survey, insights, and generalizations. J. Mach. Learn. Res. 16: 2859-2900 (2015) - [j5]Elad Gilboa, Yunus Saatci, John P. Cunningham:
Scaling Multidimensional Inference for Structured Gaussian Processes. IEEE Trans. Pattern Anal. Mach. Intell. 37(2): 424-436 (2015) - [j4]Josh Merel, Donald M. Pianto, John P. Cunningham, Liam Paninski:
Encoder-Decoder Optimization for Brain-Computer Interfaces. PLoS Comput. Biol. 11(6) (2015) - [c18]Yuanjun Gao, Lars Buesing, Krishna V. Shenoy, John P. Cunningham:
High-dimensional neural spike train analysis with generalized count linear dynamical systems. NIPS 2015: 2044-2052 - [c17]Jacob R. Gardner, Gustavo Malkomes, Roman Garnett, Kilian Q. Weinberger, Dennis L. Barbour, John P. Cunningham:
Bayesian Active Model Selection with an Application to Automated Audiometry. NIPS 2015: 2386-2394 - [c16]Jacob R. Gardner, Xinyu Song, Kilian Q. Weinberger, Dennis L. Barbour, John P. Cunningham:
Psychophysical Detection Testing with Bayesian Active Learning. UAI 2015: 286-295 - [i3]Stephan Mandt, Florian Wenzel, Shinichi Nakajima, John P. Cunningham, Christoph Lippert, Marius Kloft:
Sparse Estimation in a Correlated Probit Model. CoRR abs/1507.04777 (2015) - [i2]Josh Merel, David E. Carlson
, Liam Paninski, John P. Cunningham:
Neuroprosthetic decoder training as imitation learning. CoRR abs/1511.04156 (2015) - 2014
- [c15]Jacob R. Gardner, Matt J. Kusner, Zhixiang Eddie Xu, Kilian Q. Weinberger, John P. Cunningham:
Bayesian Optimization with Inequality Constraints. ICML 2014: 937-945 - [c14]Lars Buesing, Timothy A. Machado, John P. Cunningham, Liam Paninski:
Clustered factor analysis of multineuronal spike data. NIPS 2014: 3500-3508 - [c13]Andrew Gordon Wilson, Elad Gilboa, John P. Cunningham, Arye Nehorai:
Fast Kernel Learning for Multidimensional Pattern Extrapolation. NIPS 2014: 3626-3634 - 2013
- [c12]Elad Gilboa, Yunus Saatçi, John P. Cunningham:
Scaling Multidimensional Gaussian Processes using Projected Additive Approximations. ICML (1) 2013: 454-461 - [i1]Andrew Gordon Wilson, Elad Gilboa, Arye Nehorai, John P. Cunningham:
GPatt: Fast Multidimensional Pattern Extrapolation with Gaussian Processes. CoRR abs/1310.5288 (2013) - 2012
- [j3]Mengyuan Zhao, Aaron P. Batista
, John P. Cunningham, Cynthia A. Chestek, Zuley Rivera-Alvidrez, Rachel S. Kalmar, Stephen I. Ryu, Krishna V. Shenoy, Satish Iyengar:
An L 1-regularized logistic model for detecting short-term neuronal interactions. J. Comput. Neurosci. 32(3): 479-497 (2012) - [c11]Vikash Gilja, Paul Nuyujukian, Cindy A. Chestek, John P. Cunningham, Byron M. Yu, Joline M. Fan, Stephen I. Ryu, Krishna V. Shenoy:
A brain machine interface control algorithm designed from a feedback control perspective. EMBC 2012: 1318-1322 - [c10]John P. Cunningham, Zoubin Ghahramani, Carl Edward Rasmussen:
Gaussian Processes for time-marked time-series data. AISTATS 2012: 255-263 - 2011
- [c9]Biljana Petreska, Byron M. Yu, John P. Cunningham, Gopal Santhanam, Stephen I. Ryu, Krishna V. Shenoy, Maneesh Sahani:
Dynamical segmentation of single trials from population neural data. NIPS 2011: 756-764 - [c8]Jakob H. Macke, Lars Buesing, John P. Cunningham, Byron M. Yu, Krishna V. Shenoy, Maneesh Sahani:
Empirical models of spiking in neural populations. NIPS 2011: 1350-1358
2000 – 2009
- 2009
- [j2]Catie Chang, John P. Cunningham, Gary H. Glover:
Influence of heart rate on the BOLD signal: The cardiac response function. NeuroImage 44(3): 857-869 (2009) - [j1]John P. Cunningham, Vikash Gilja, Stephen I. Ryu, Krishna V. Shenoy:
Methods for estimating neural firing rates, and their application to brain-machine interfaces. Neural Networks 22(9): 1235-1246 (2009) - [c7]Matthias W. Seeger, Suvrit Sra
, John P. Cunningham:
Workshop summary: Numerical mathematics in machine learning. ICML 2009: 9 - 2008
- [c6]John P. Cunningham, Krishna V. Shenoy, Maneesh Sahani:
Fast Gaussian process methods for point process intensity estimation. ICML 2008: 192-199 - [c5]Byron M. Yu, John P. Cunningham, Gopal Santhanam, Stephen I. Ryu, Krishna V. Shenoy, Maneesh Sahani:
Gaussian-process factor analysis for low-dimensional single-trial analysis of neural population activity. NIPS 2008: 1881-1888 - 2007
- [c4]Byron M. Yu, John P. Cunningham, Krishna V. Shenoy, Maneesh Sahani:
Neural Decoding of Movements: From Linear to Nonlinear Trajectory Models. ICONIP (1) 2007: 586-595 - [c3]John P. Cunningham, Byron M. Yu, Krishna V. Shenoy, Maneesh Sahani:
Inferring Neural Firing Rates from Spike Trains Using Gaussian Processes. NIPS 2007: 329-336 - 2006
- [c2]John P. Cunningham, Byron M. Yu, Krishna V. Shenoy:
Optimal Target Placement for Neural Communication Prostheses. EMBC 2006: 2912-2915 - [c1]Krishna V. Shenoy, Teresa H. Santhanam, Stephen I. Ryu, Afsheen Afshar, Byron M. Yu, Vikash Gilja, Michael D. Linderman, Rachel S. Kalmar, John P. Cunningham, Caleb T. Kemere
, Aaron P. Batista
, Mark M. Churchland, Teresa H. Meng:
Increasing the Performance of Cortically-Controlled Prostheses. EMBC (Supplement) 2006: 6652-6656
Coauthor Index

manage site settings
To protect your privacy, all features that rely on external API calls from your browser are turned off by default. You need to opt-in for them to become active. All settings here will be stored as cookies with your web browser. For more information see our F.A.Q.
Unpaywalled article links
Add open access links from to the list of external document links (if available).
Privacy notice: By enabling the option above, your browser will contact the API of unpaywall.org to load hyperlinks to open access articles. Although we do not have any reason to believe that your call will be tracked, we do not have any control over how the remote server uses your data. So please proceed with care and consider checking the Unpaywall privacy policy.
Archived links via Wayback Machine
For web page which are no longer available, try to retrieve content from the of the Internet Archive (if available).
Privacy notice: By enabling the option above, your browser will contact the API of archive.org to check for archived content of web pages that are no longer available. Although we do not have any reason to believe that your call will be tracked, we do not have any control over how the remote server uses your data. So please proceed with care and consider checking the Internet Archive privacy policy.
Reference lists
Add a list of references from ,
, and
to record detail pages.
load references from crossref.org and opencitations.net
Privacy notice: By enabling the option above, your browser will contact the APIs of crossref.org, opencitations.net, and semanticscholar.org to load article reference information. Although we do not have any reason to believe that your call will be tracked, we do not have any control over how the remote server uses your data. So please proceed with care and consider checking the Crossref privacy policy and the OpenCitations privacy policy, as well as the AI2 Privacy Policy covering Semantic Scholar.
Citation data
Add a list of citing articles from and
to record detail pages.
load citations from opencitations.net
Privacy notice: By enabling the option above, your browser will contact the API of opencitations.net and semanticscholar.org to load citation information. Although we do not have any reason to believe that your call will be tracked, we do not have any control over how the remote server uses your data. So please proceed with care and consider checking the OpenCitations privacy policy as well as the AI2 Privacy Policy covering Semantic Scholar.
OpenAlex data
Load additional information about publications from .
Privacy notice: By enabling the option above, your browser will contact the API of openalex.org to load additional information. Although we do not have any reason to believe that your call will be tracked, we do not have any control over how the remote server uses your data. So please proceed with care and consider checking the information given by OpenAlex.
last updated on 2024-10-07 21:18 CEST by the dblp team
all metadata released as open data under CC0 1.0 license
see also: Terms of Use | Privacy Policy | Imprint